The Ultimate Guide to Data Labelling Tools: Unleashing the Power of Data Annotation
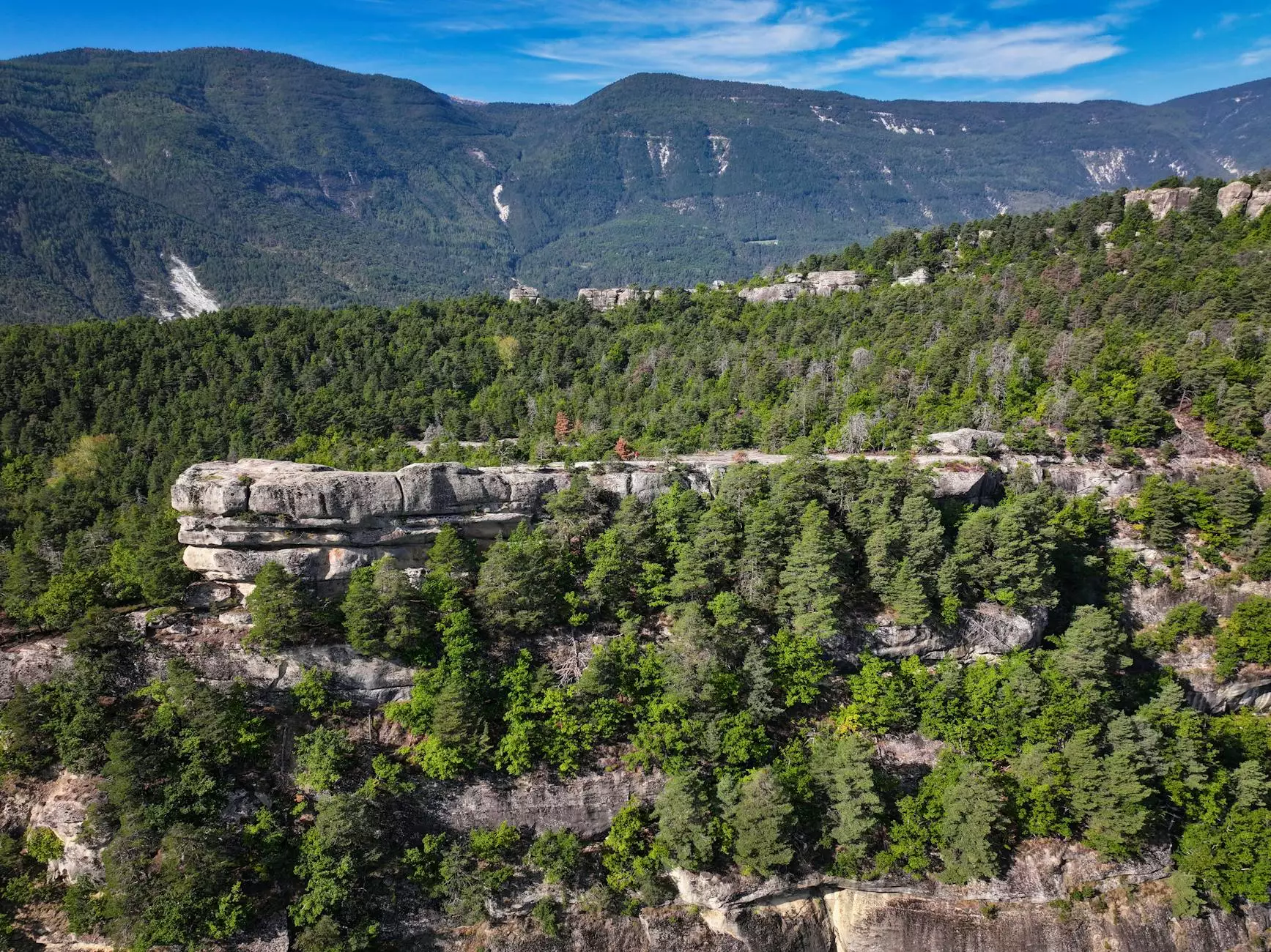
In today's fast-paced digital environment, data is king. Organizations across various industries are increasingly recognizing the value of harnessing data to drive decision-making and strategic planning. However, for data to be truly valuable, it must be properly labelled and annotated. This is where a data labelling tool becomes crucial. In this comprehensive guide, we will explore data labelling, its importance, various types of annotation tasks, and how platforms like keylabs.ai can enhance your data annotation workflow.
Understanding Data Labelling
Data labelling is the process of identifying and tagging data with relevant information. This technique is essential for training machine learning models, enabling them to recognize patterns and make predictions. Whether it’s images, text, or audio, labelled data is instrumental in creating accurate AI systems. The effective use of a data labelling tool ensures that your data is organized and understood, leading to more reliable outcomes.
Why Data Labelling is Critical for Businesses
As organizations invest in AI and machine learning technologies, the need for high-quality, labelled datasets has grown exponentially. Here are a few reasons why data labelling is critical:
- Improved Model Accuracy: Labelled data provides the foundation for training machine learning models, leading to better accuracy and performance.
- Scalability: Automated data labelling tools can handle large volumes of data efficiently, saving time and resources.
- Customization: Different businesses have unique requirements. Tailored data labelling solutions can cater to specific industry needs and ensure relevant data is used.
- Time Efficiency: By using a robust data annotation platform, organizations can accelerate the process of data preparation, allowing them to focus on analysis.
Types of Data Labelling Tasks
Data labelling encompasses a variety of annotation tasks, each serving unique purposes:
1. Image Annotation
Image annotation involves adding labels to images to identify objects or features within them. Common techniques include:
- Bounding Boxes: Enclosing objects in rectangular boxes to identify their location.
- Semantic Segmentation: Classifying each pixel in an image to provide detailed breakdowns of scenes.
- Keypoint Annotation: Marking specific points of interest on an object for pose estimation.
2. Text Annotation
Text annotation refers to labeling parts of written text for various applications. This can involve:
- Named Entity Recognition (NER): Identifying and classifying elements in text into predefined categories.
- Sentiment Analysis: Classifying the emotions expressed in a text as positive, negative, or neutral.
- Topic Tagging: Assigning tags or categories to text based on its content.
3. Audio Annotation
Audio annotation entails labelling elements within audio files. Key types of audio tasks include:
- Speech Recognition: Transcribing spoken language into written text.
- Sound Event Detection: Identifying specific sounds and tagging them within an audio file.
The Benefits of Using a Data Labelling Tool
Leveraging a data labelling tool is essential for maximizing the effectiveness of your data annotation process. Here are some significant benefits:
- Enhanced Productivity: Automating the data labelling process significantly speeds up workflow, allowing teams to focus on higher-level analysis.
- Quality Assurance: Many data labelling platforms include features to ensure the quality and accuracy of labels, mitigating errors that can skew results.
- Collaboration Capabilities: Advanced platforms support team collaboration, enabling multiple members to work on projects simultaneously, streamlining the process.
- Integration with Machine Learning Models: The right tool can seamlessly integrate with your existing machine learning workflows, improving productivity.
KeyLabs.ai: Pioneering Data Annotation Solutions
Among the myriad of data annotation platforms available, keylabs.ai stands out for its innovative approach and comprehensive features. KeyLabs.ai offers a suite of tools designed to cater to businesses' unique needs, enhancing their ability to prepare data for machine learning effectively.
Why Choose KeyLabs.ai?
KeyLabs.ai provides a customizable data labelling tool that ensures precision and accuracy in data annotation. Here are some compelling reasons to consider their platform:
- User-Friendly Interface: The intuitive design of KeyLabs.ai allows users to quickly adapt and utilize the tool effectively, regardless of technical proficiency.
- Advanced Annotation Options: With various annotation techniques, including bounding box, polygon and landmark annotations, you can cater to specific project requirements.
- Automated Labelling Features: KeyLabs.ai utilizes AI to facilitate automated labelling, saving time and resources.
- Scalable Solutions: Whether you’re a startup or a large enterprise, KeyLabs.ai’s solutions are designed to scale alongside your business needs.
Integrating Your Data Labelling Tool into Business Workflows
Successfully integrating a data labelling tool into your business processes requires a strategic approach. Here are critical steps to consider:
1. Assess Your Annotation Needs
Evaluate the types of data you are working with and identify the specific annotation tasks required. Understanding your project scope will help you select a platform that meets your needs.
2. Choose the Right Tool
After assessing your requirements, choose a tool that offers the functionalities you need while being user-friendly and scalable.
3. Train Your Team
Ensure that your team is familiar with the platform and its features. Quality training can significantly enhance productivity and data quality.
4. Monitor and Evaluate
As you implement your data labelling strategy, continuously monitor the process and evaluate results. Adjust workflows and practices as necessary to optimize efficiency.
Future Trends in Data Labelling
The field of data labelling is evolving rapidly, and staying updated with future trends is essential for businesses looking to maintain a competitive edge. Here are some predicted trends:
- Increased Automation: The future will see more advanced AI techniques to automate data labelling processes, reducing the need for manual intervention.
- Focus on Annotated Data Quality: Organizations are likely to prioritize the quality of labelled data over quantity, ensuring better model performance.
- Integration of Crowd-sourcing: Leveraging human intelligence for complex labelling tasks will continue to be an important strategy, especially for nuanced data.
- Real-Time Annotation Tools: The introduction of real-time data labelling tools will streamline collaboration and enhance productivity across teams.
Conclusion
In conclusion, utilizing a data labelling tool is paramount for businesses aiming to leverage the power of data in today's competitive environment. With platforms like keylabs.ai, effectively annotating your data has never been easier. By investing in quality data labelling, your organization can unlock the potential of your datasets, driving innovation and improving decision-making processes. The significance of high-quality labelled data will continue to grow, making it essential for businesses to adopt robust data annotation strategies now and into the future.